Full-text Search in Django with Postgres and Elasticsearch
- Updated May 22nd, 2023
- v3.0.0
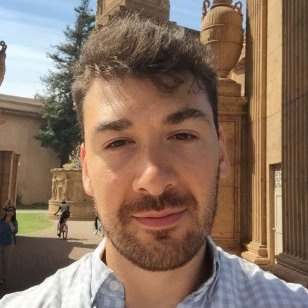
Learn how to add full-text search to Django with both Postgres and Elasticsearch!
In this course, you'll learn how to craft a search application that helps users peruse a large catalog of wines. We'll start by building a simple Django project using Docker. Then, we'll do a deep dive into the native search capabilities offered by Postgres, and we'll incorporate them into our app using both the Django library and our own hand rolled constructs. Next, we'll create a React app to drive the user experience. Finally, we'll take our search app to the next level and swap out Postgres for Elasticsearch. And of course we'll be using Test-driven Development every step of the way.
What will you learn?
Select a Part
In Part 1, you'll learn how to program the server code of the search application. We'll start by developing a basic Django app using a Postgres database in Docker. We'll create the basic data model, code the API, and write tests.
Learning Objectives
- Describe a high-level system design to a friend or colleague
- Create a basic Django app that uses a RESTful API
- Containerize an app using Docker
- Understand basic Django REST Framework concepts (serializers, filters, views, etc.)
- Paginate an API
- Practice Test-driven Development (TDD)
In Part 2, you'll explore Postgres queries while incorporating them into the search application. We'll go step-by-step in transforming the foundational app we created in Part 1 into a robust search service.
We'll start by learning the fundamentals of full-text search, and then we'll slowly build the concepts into our API and demonstrate how they improve the search results. You'll learn how to use database indexes to boost performance, rank results by relevancy, highlight keywords, and offer suggestions for misspelled words. By the end of Part 2, you'll have a good handle on how to write raw SQL queries and the companion Django code.
Learning Objectives
- List some ways that full-text search matches a user's query better than simple alternatives
- Implement full-text search in a Django app using a Postgres database
- Sort full-text search results by relevancy
- Update a data model to improve full-text search performance
- Highlight full-text search matches
- Make suggestions to a user who searches for a misspelled word
In Part 3, you'll code a React app that provides a user interface for the APIs you built in Parts 1 and 2. The UI is broken into two components: a search form and a results list. Along the way, we'll write end-to-end tests using Cypress.
Learning Objectives
- Create a React app using Create React App
- Build functional forms with Formik
- Communicate with the server through HTTP requests
- Code a React component to fetch and display paginated results
- Test an application end-to-end using Cypress
- Build a production version of a React app
In Part 4, you'll learn how to use Elasticsearch in place of Postgres to provide comparable functionality. We'll explore how to replicate the same search techniques, such as relevancy boosting and suggestions, using Elasticsearch's concepts and Query DSL.
We'll start by configuring an Elasticsearch cluster with a single node in Docker. Next, we'll build an index and walk through how to add features and what affects they have on the search results, just like we did in Part 2 for Postgres. We'll cap things off by using Nginx to serve traffic between the frontend and the backend.
Learning Objectives
- Configure Elasticsearch for local development
- Create an Elasticsearch index and populate it with documents
- Perform searches using Query DSL
- Highlight search matches
- Make suggestions to a user who searches for a misspelled word
What do you need to know?
This is not a beginner course. It's designed for the advanced-beginner -- someone with at least six months of web development experience. Before beginning, you should have some familiarity with the following topics. Refer to these resources for more info:
Django
- Test-Driven Development with Python, Django, and Docker
- Looking for a solid introduction to Django? Check out Django for Beginners. Highly recommended!
React
What developers are saying
The TestDriven.io courses are some of the best courses I've ever done for any language, any platform, any price range... just some of the most thorough and well-sourced courses around.
Just a word of thanks for doing such a great job with these training courses. The thorough, entire-lifecycle approach -- from implementation through test, coverage, quality, CI/CD, and all the rest -- is what separates these courses from other training material that I've completed. I'll be able to walk away from here with knowledge and skills that I can apply immediately at work -- and for that I'm grateful. It's a rare gift in an environment where so much 'training' is really just lightweight treatment that doesn't begin to scratch the surface of real, end-to-end software development. Really well done!
The TestDriven.io courses are worth 10 times what I paid for them.
I'm writing to thank you for all the tutorials and the work you've put out there. I'm new to DevOps and I found TestDriven.io while looking for Django and DevOps related topics. One of the best collections of tutorials and guides I've seen -- very well-written, clear, and concise. You have saved me so much time and energy. Thanks from the bottom of my heart.
I am very much into buying and purchasing any course by you and your team. I've never felt like a better programmer ready to show my coding chops to the world.
Frequently Asked Questions
What will you build?
Django backend: We'll construct a RESTful API with Django REST Framework that communicates with both Postgres and Elasticsearch data sources
React frontend: We'll build a React app that lets users search a catalog of 150,000+ wines and displays their results
Postgres database: We'll make a data model, explore SQL queries, and learn techniques to help us boost performance
Elasticsearch: We'll create an index, tweak a mapping, and learn how to use the Query DSL
What tools and technologies are used in this course?
This course covers a variety of technologies and services:
Back-end
- Python
- Django
- Django REST Framework
- Django Channels
- Postgres
- Elasticsearch
- Gunicorn
Front-end
- JavaScript
- React
- Cypress
Tools
- Docker
- Nginx
What support does TestDriven.io offer?
Since the courses mimic real-world development, support is provided via Stack Overflow. Helpful users, including the developers of the courses, read and respond to messages on Stack Overflow. If you get stuck and you can't find an answer via Stack Overflow, feel free to reach out via email directly. Just be sure to detail what you've tried. For more, review Support and Consulting.
How long does it take to complete the course?
It's dependent on your current skill level. On average, it takes approximately 12 hours to complete.